Predictive Maintenance: Enhancing Energy Infrastructure Reliability
- Rohini Thakur
- Apr 3, 2023
- 4 min read
In the complex and ever-evolving world of energy, reliability is paramount. Whether it's a power plant generating electricity, an oil refinery producing fuels, or a wind farm harnessing renewable energy, the equipment and infrastructure that make it all possible must operate efficiently and reliably. Unplanned downtime is not just an inconvenience; it can result in significant financial losses and, in some cases, pose safety risks. This is where Artificial Intelligence (AI) and predictive maintenance come into play.
Predictive maintenance is a cutting-edge approach that uses AI and data analysis to predict when equipment is likely to fail. By proactively identifying potential issues and scheduling maintenance activities before they become critical, companies can save money, reduce downtime, and improve overall operational efficiency. In the energy sector, the implications are profound.
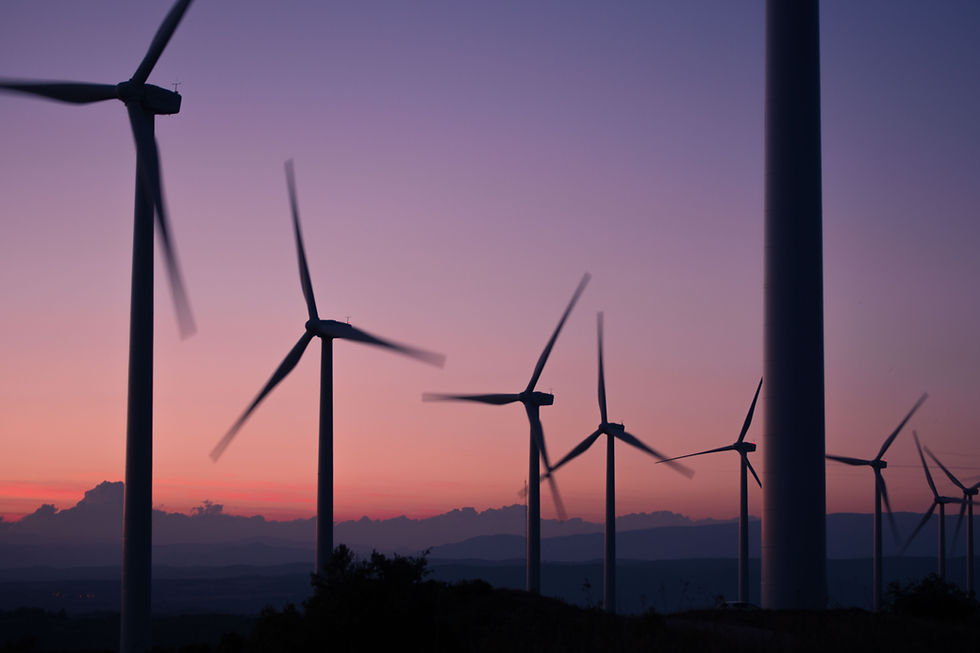
The State of Energy Infrastructure
The energy sector is diverse, encompassing various industries, from oil and gas to renewable energy. However, a common thread among all these industries is the critical need for infrastructure reliability. Any unplanned downtime can result in substantial financial losses and, in some cases, environmental damage or even public safety concerns.
Traditionally, maintenance has been a reactive process. When equipment breaks down or malfunctions, maintenance crews are dispatched to fix the problem. While this approach can address immediate issues, it has several drawbacks. It often results in higher maintenance costs, as urgent repairs can be more expensive than routine maintenance. Moreover, downtime can lead to lost productivity and, in the energy sector, the loss of revenue from interrupted production.
The Predictive Maintenance Revolution
Predictive maintenance, enabled by AI and advanced data analytics, represents a paradigm shift in maintenance strategies. Instead of waiting for equipment to fail, predictive maintenance uses historical data, sensor information, and AI algorithms to identify early warning signs of potential issues. These warning signs are detected long before a breakdown occurs, allowing maintenance teams to schedule interventions at a time that minimizes disruption.
Key Elements of Predictive Maintenance:
Data Collection: Predictive maintenance relies on extensive data collection. Sensors placed on equipment continuously monitor various parameters, such as temperature, pressure, vibration, and more.
Data Analysis: AI algorithms analyze the collected data to identify patterns and anomalies. These algorithms can detect subtle changes that may indicate impending failures.
Predictive Models: AI models are used to predict when maintenance is needed. These models consider various factors, such as historical data, environmental conditions, and equipment age.
Condition-Based Alerts: Predictive maintenance systems generate alerts and recommendations for maintenance actions. These alerts can be used to schedule maintenance at the most convenient time, ensuring minimal disruption.
Benefits in the Energy Sector
The energy sector stands to benefit significantly from the adoption of predictive maintenance. Here are some of the key advantages:
1. Reduced Downtime: Predictive maintenance helps energy companies avoid unscheduled downtime. By fixing issues before they become critical, facilities can operate continuously, ensuring a steady supply of energy.
2. Cost Savings: While there is an upfront investment in implementing predictive maintenance systems, the long-term cost savings are substantial. By addressing problems proactively, companies can avoid costly emergency repairs and extend the lifespan of equipment.
3. Improved Safety: Preventing unexpected equipment failures enhances safety in the energy sector. This is especially critical in industries like oil and gas, where accidents can have catastrophic consequences.
4. Enhanced Efficiency: Predictive maintenance optimizes the use of resources by focusing on the most critical maintenance tasks. This reduces unnecessary interventions and minimizes disruptions to operations.
5. Environmental Benefits: In renewable energy, predictive maintenance can help maximize the uptime of clean energy sources like wind turbines and solar panels, reducing the reliance on fossil fuels and lowering greenhouse gas emissions.
Real-World Applications
Predictive maintenance is not just a theoretical concept; it is being actively deployed in the energy sector with impressive results. Let's look at a few real-world examples:
1. Wind Turbine Maintenance: Wind farms are often located in remote areas, making maintenance challenging and costly. Predictive maintenance uses sensors on wind turbines to monitor performance. By analyzing data on factors such as wind speed, temperature, and equipment vibration, maintenance teams can predict when a component is likely to fail. This enables scheduled maintenance, reducing downtime and repair costs.
2. Oil Refinery Equipment: In the oil and gas industry, refineries rely on a vast array of equipment, from pumps to heat exchangers. Any unplanned downtime can be financially crippling. Predictive maintenance helps by monitoring the condition of these critical assets. If a pump starts to show signs of vibration or temperature anomalies, the system can alert the maintenance team. Maintenance can be scheduled during a planned downtime period, avoiding costly emergency repairs.
3. Power Plant Operations: Power plants, whether they use fossil fuels or renewable sources, require precise monitoring and maintenance. Predictive maintenance is particularly crucial in preventing costly breakdowns and optimizing equipment performance. By analyzing data from turbines, generators, and other critical components, power plants can schedule maintenance during periods of low demand, reducing the impact on electricity supply.
The Challenges of Predictive Maintenance
While predictive maintenance offers numerous benefits, it's not without challenges:
Data Quality: Predictive maintenance relies on high-quality data. If sensors malfunction or data is inaccurate, the system's predictions may be unreliable.
Initial Investment: Implementing a predictive maintenance system requires an initial investment in sensors, data infrastructure, and AI software.
Data Security: Protecting sensitive equipment and operational data is critical. Ensuring data security and privacy is a significant concern in predictive maintenance.
Employee Training: Training staff to work with predictive maintenance systems and interpret their recommendations is essential for success.
Integration: Integrating predictive maintenance systems with existing infrastructure and processes can be complex.
Algorithm Accuracy: The accuracy of predictive models depends on the quality of the data and the design of the algorithms. Continuous improvement is necessary.
Conclusion
In the energy sector, where reliability and efficiency are paramount, predictive maintenance powered by AI is a game-changer. It offers the ability to prevent costly breakdowns, reduce downtime, and extend the lifespan of critical equipment. With the potential to save substantial sums of money and enhance safety, the adoption of predictive maintenance in the energy sector is a logical and necessary step toward a more reliable and sustainable energy future. As technology continues to advance, we can expect even more sophisticated predictive maintenance solutions, further solidifying its role as an indispensable tool in the energy industry.
Comentarios